Verifiable Generation with Subsentence-Level Fine-Grained Citations
Shuyang Cao and Lu Wang (Finding of ACL 2024)
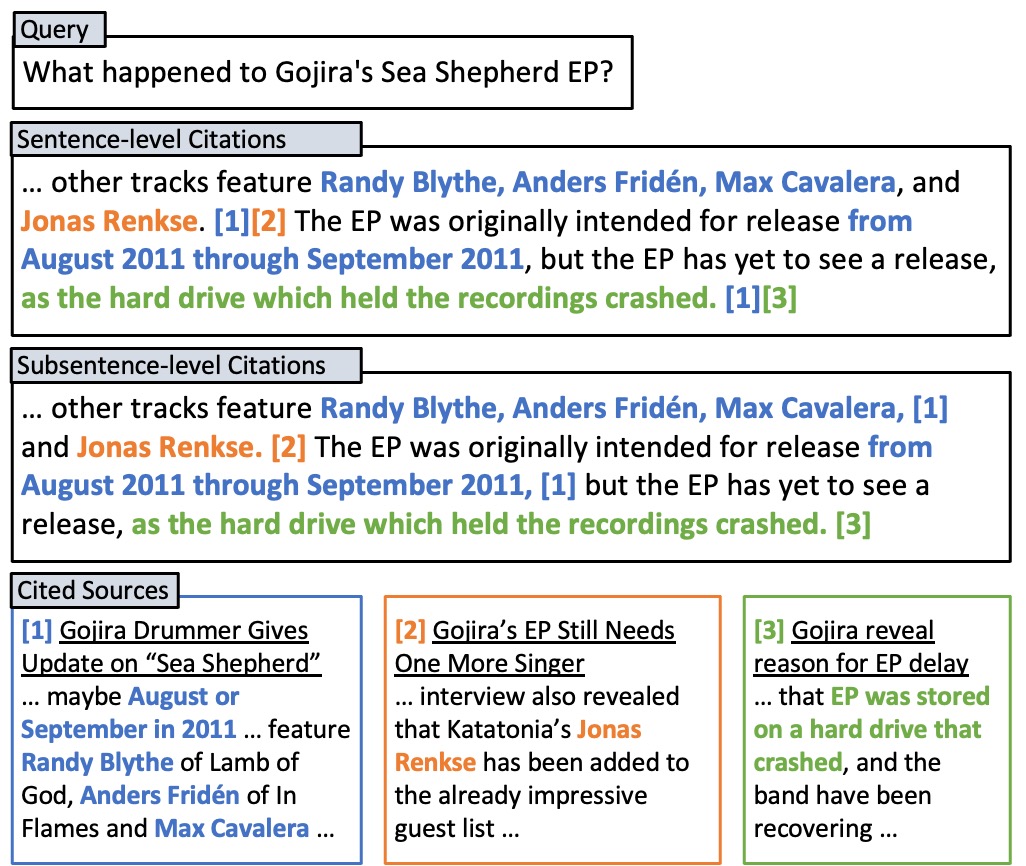
Abstract:
Verifiable generation requires large language models (LLMs) to cite source documents supporting their outputs, thereby improve output transparency and trustworthiness. Yet, previous work mainly targets the generation of sentence-level citations, lacking specificity about which parts of a sentence are backed by the cited sources. This work studies verifiable generation with subsentence-level fine-grained citations for more precise location of generated content supported by the cited sources. We first present a dataset, SciFi, comprising 10K Wikipedia paragraphs with subsentence-level citations. Each paragraph is paired with a set of candidate source documents for citation and a query that triggers the generation of the paragraph content. On SciFi, we evaluate the performance of state-of-the-art LLMs and strategies for processing long documents designed for these models. Our experiment results reveals key factors that could enhance the quality of citations, including the expansion of the source documents’ context accessible to the models and the implementation of specialized model tuning.
Data:
The usage of the data is restricted by the licenses and copyright policies of the original data sources (if any).